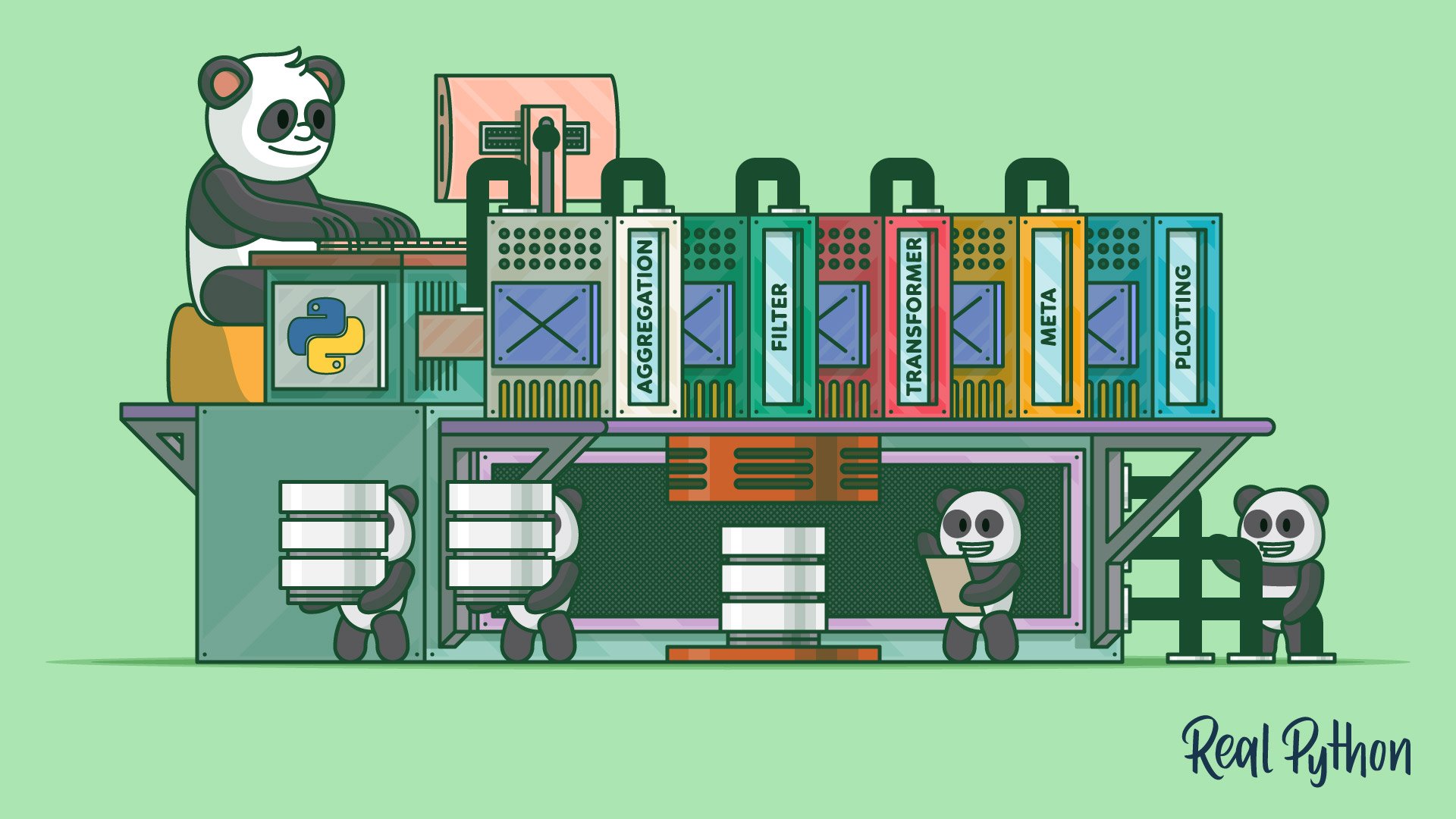
이번 포스팅에서는 Pandas series의 method에 대한 내용을 정리해보고자 한다.
Pandas series의 method
1 | import pandas as pd |
Pandas 활용해서 CSV 파일 읽기
1 | #Pandas의 read_csv method를 활용해서 csv파일을 읽기 위해서는 squeeze=True option은 필수이다. |
위의 각 결과를 보면 알 수 있듯이, Pandas series의 경우에는 formatting 없이 단순하게 (1차원의)하나의 열로 테이블화 된 형태로 데이터가 출력이 되고, DataFrame의 경우에는 각 셀에 스타일이 적용되어 테이블이 formatting되어 데이터가 출력된다.데이터 프레임(DataFrame)이란 1개의 열이 아닌, 여러 행과 열을 가진 다차원 데이터 테이블
이다.
Pandas 빌트인 함수
Pandas는 이미 존재하는 python의 수 많은 함수들과 상호동작한다.
1 | sp500 = pd.read_csv('S&P500_Prices.csv', squeeze=True) |
Pandas series sorting(ascending order)
1 | import pandas as pd |
Pandas Series Math operation
1 | sp500 = pd.read_csv('S&P500_Prices.csv', squeeze=True) |